Trustworthy AI Experiences
Explore tools and inspiration around explaining how AI mechanisms work, through hands-on prototyping, testing and iterating towards implementation.
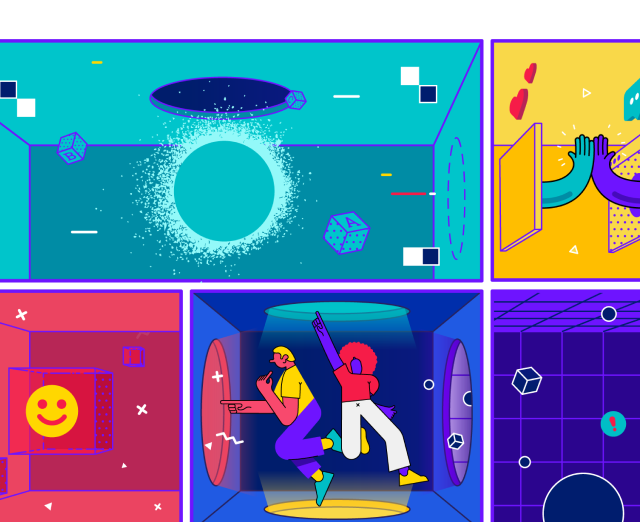
AI-driven products and systems are becoming increasingly widespread. More people are interacting with AI every day, knowingly or otherwise. As we progress, we should continue to develop processes, governance frameworks, and regulations to ensure that AI systems are as trustworthy as possible. We also need to empower people to decide for themselves whether to trust an AI system in any given context.
We believe that there are at least three approaches to help with this:
1. Help people to better understand AI, by explaining when and how they are interacting with an AI system, and being transparent about the decisions it makes and the outcomes it produces;
2. Give people meaningful control over their relationship with an AI system, giving them choices and agency where it makes sense to do so;
3. Give people confidence that there are checks and balances in place, much like in other parts of society, by developing processes and mechanisms of accountability.
TTC Labs has been bringing together industry, policymakers and experts in an effort to understand how we can develop more sustainable relationships between AI-driven products and the people who use them.
Learn MoreUnderstanding AI
How can we empower people to make informed decisions about trusting an AI system?
Helping people to Understand AI
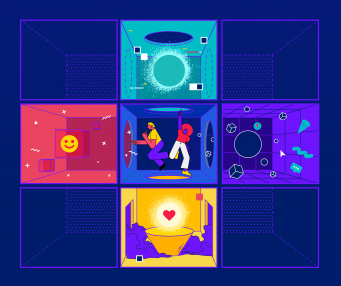
When people don’t understand how an AI-driven service comes up with results, they may feel less able to discern whether or not to trust it. We therefore need to design mechanisms to explain to people when, how and why they are affected by AI systems, to help support greater comprehension of AI.
These mechanisms should drive practical understanding and agency for everybody, not just for the narrow few. That means the explanations must be provided in a way that caters for non-experts, and gives people the information they need to make a reasonably informed judgement.
The goal is not product explanations, but people’s understanding.
People are not passive recipients of AI explanations.
Antonio

“I like being able to open a new app and just understand how to use it, without long instructions.”
Through our interactive workshops and research, we’ve found that people draw on their wider knowledge and experience to develop their understanding of an AI in an active manner. Their understanding can change depending on how a product behaves and surfaces results, as people situate explanations within the context of their experience. This means that we must adopt a more interactive concept of explainability, moving beyond the idea that product makers provide explanations and product users merely receive them. Product makers can take a more collaborative approach to explainability through the use of interactive touchpoints, user controls and implicit explainability mechanisms.
Not everyone requires the same level of information
Rochelle
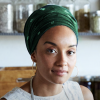
“I don't know why some articles are surfaced over others, but I'm ok with that.”
Comprehensive transparency and highly detailed explanations aren’t always useful, desired, or even possible. The key to creating positive user experiences is knowing how much to reveal and when.
Product makers need to ensure people are provided with the information most relevant to their respective needs and contexts. They also need to consider how explainability is balanced between different audiences, such as general product users and expert stakeholders, and acknowledge the trade-offs that come with this. When a product serves different user groups, the information and control appropriate to each can vary significantly.
It also means accounting for the fact that different people engage with information in different ways, by designing multiple opportunities for a user to develop understanding in a way that is meaningful to them.
Further Reading
In Control of AI
Control is about giving people meaningful agency over their relationship with an AI-powered system.
Empowering People with Control
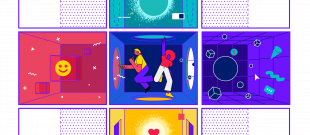
Controls take many forms; they include mechanisms that allow people to influence the way an AI-powered product generates results, to modify their declared interests and preferences, or to override or remove AI-inferred data inputs. They also include opportunities for people to provide feedback on recommendations and to challenge AI outcomes and explanations if they disagree with them.
Comprehensive control is not necessarily possible or desirable, but appropriate controls can enhance people’s understanding of AI systems. There is more work to be done to identify and develop user controls that can improve transparency and explainability of AI systems for different audiences.
Control requires meaningful agency over AI interactions
Rochelle

“When there are tons of pop-ups or big blocks of text, I just click through.
Control is about empowering people over their interactions with AI systems. The relative complexity of AI means explaining how and why a system uses information, such as someone’s personal data, is not always straightforward.
And giving people a lot of information about how their data might be used is not the same as meaningful control – it outsources responsibility to the user, instead of the product or system maker.
Meaningful agency means control over inputs, and the ability to feedback into AI processes. It may also include a choice of outputs of AI systems.
Are you working in this space?
We'd love to hear from you.
Our explorations on this topic are just a beginning. These challenges require more work, and more insight from the wider community.
Get in TouchInitiated and supported by Meta, TTC Labs is an experimental data design initiative.
TRUSTWORTHY AI EXPERIENCES
Accountability
Accountability focuses on tracing and verifying system outputs and actions. It involves being clear about which parts of a system a person is accountable for and to whom they are accountable.
Transparency Strengthens Accountability
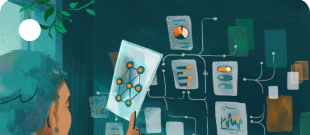
System Cards, a new resource for understanding how AI systems work
Learn MoreTechnical documentation, such as model or system cards, can provide insights into why and for what purpose an AI model or system was developed, how it was trained, how it produces results and how it will evolve over time.
By producing this documentation product makers are given an opportunity to reflect on their processes which promotes accountability within an organisation, and allowing external experts to verify it can provide assurances to non-experts that the necessary oversight mechanisms are in place, and working effectively.
With adequate information, and clear routes to redress, users are empowered to make an informed decision about the AI systems they use