Authors’ note: Product teams at Facebook rely on research along with other external factors to design and build products. This article discusses research conducted by Facebook's Privacy Research Team to better understand people's privacy concerns.
Abstract
Traditional ways of measuring users’ privacy concerns can produce ambiguous results that are difficult to take action on in applied settings.
The Privacy Beliefs and Judgments (PB&J) framework is a new approach to measuring privacy concerns that addresses these limitations. It’s flexible and can be adapted to measure privacy concerns for a variety of topics and products.
Report
If you wanted to measure privacy concerns for a product or experience, how would you do it? A common approach is to ask a straightforward question like “How concerned are you about privacy on [that app]?” Unfortunately, there are two reasons why this simple question isn’t nearly as insightful or actionable as it might seem.
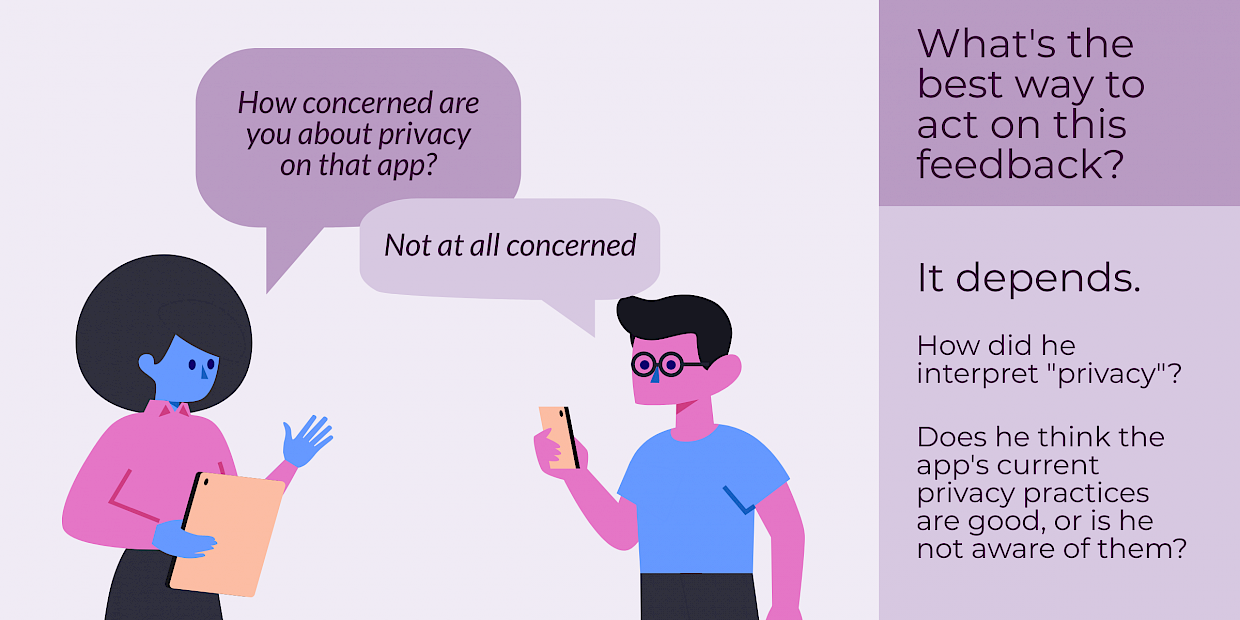
First, “privacy” is an ambiguous term that can refer to many things. When someone says they’re concerned about privacy, they might be expressing concern about who can see photos and other content they’ve shared online, about what information an app or site collects about them, about whether strangers can send them direct messages, or perhaps something else. In fact, previous research found 16 distinct privacy concerns that people have for online experiences (Hepler & Blasiola, 2021). So when you ask people how concerned they are about privacy and they say “somewhat or very concerned”, it’s unclear how you can or should act on that information because you don’t know which specific privacy topic(s) caused their concern. Therefore, to make privacy concern measures more actionable, they need to ask about specific privacy topics. For example: “How concerned are you about [this app] using information about you to determine what ads to show you on [this app]?”
A second issue is that “concern” itself can be ambiguous. If someone responds “very concerned” to the question above, the implications are clear - they believe this could happen and that it would be undesirable for it to happen. However, what if they say “not concerned”? That could reflect two distinct circumstances:
1. They believe it could happen, but think it would be desirable or neutral.
2. They don’t believe it would happen, regardless of how (un)desirable it would be.
Unfortunately, when questions simply ask people to report “how concerned” they are about privacy, it inherently creates this ambiguity and makes responses less actionable than they need to be. For example, if people are “not concerned” about Facebook using information about them to determine what ads to show them on Facebook, should Facebook take that as a signal that it’s ok to do so? Or should Facebook take that as a signal that people don’t believe it’s currently happening, but might not be ok with it if they thought it was?
Privacy beliefs & judgments: A way to improve the actionability of privacy concern measures
Due to the limitations of simple privacy concern questions, Facebook’s Privacy Research Team developed a new approach to measure users’ privacy concerns. This approach has two notable elements. First, we measure perceptions for specific privacy topics rather than the overall concept of privacy. Second, we ask two questions about each topic to measure the constituent parts of concern: (a) Whether respondents believe a privacy outcome is happening, and (b) whether respondents judge that outcome to be good or bad. For example, here is how we would measure concern related to the Facebook app using information to personalize ads:
1. Do you believe Facebook uses information about you to determine what ads to show you on Facebook? [Yes, No]
2. If Facebook did that, would it be good or bad? [Good, Neither good nor bad, Bad]
We refer to this framework as the Privacy Beliefs &Judgments (PB&J) framework because the concepts we’re directly measuring are beliefs and judgments about privacy topics. This approach avoids the limitations of the direct privacy concern measures discussed above, thereby providing more actionable insights for our teams.
Importantly, we can combine the belief and judgment responses in the PB&J framework to construct a measure of privacy concern. In the sample questions above, if someone said “Yes” to question 1 and “Bad” to question 2, we could label them as being concerned about Facebook using information about them to personalize ads. Although this is an indirect measure of privacy concerns, it’s more actionable than direct measures.
Across Facebook products, researchers have been using the PB&J framework to measure privacy concerns for a variety of topics. Through testing, we’ve learned the following about the PB&J framework:
For the PB&J judgment question (“If [app] did that, would it be good or bad?”), 3-point scales provide similar results to 5-point scales. We often use 3-point scales to simplify the survey experience. Further, unipolar scales (Not at all bad, Somewhat bad, Very bad) provide similar results to bipolar scales (Good, Neither good nor bad, Bad). We often use unipolar scales for items where it may seem insensitive to imply the outcome could be good. For example, we don’t want to make it difficult for someone to remove something from their account, so we typically pair a unipolar judgment question with the following belief question: “Do you believe [app] makes it difficult for you to remove things from your [app] account that you don't want to be there?”
Through cognitive testing, we learned it’s important to include a timeframe for items that measure interpersonal privacy concerns (for a list of interpersonal privacy concerns, see Hepler & Blasiola, 2021). For example, “During the next month, do you believe anyone will add you to a group on [app] that you don't want to be added to?” Interpersonal privacy concerns often deal with one-off actions that occur at a specific point in time. When a timeframe isn’t provided, respondents tend to answer based on whether that topic ever happened to them in the past rather than whether they think it will be likely to occur in the future.
Implications
Because traditional privacy concern measures tend to be ambiguous and inactionable, we encourage organizations to adopt new ways of measuring privacy concerns that avoid the limitations discussed in this article. The PB&J framework is one approach to accomplish this goal that we’ve found valuable at Facebook, but others may exist.
To help other organizations adopt this framework, the appendix below includes a PB&J survey that we developed to measure the 16 top-of-mind user privacy concerns that we identified in previous research (Hepler & Blasiola, 2021). This survey can easily be adapted to measure concerns for other products or services.