Learning how to make policy work better for society is a continuous evolving process. As a group of Masters students in Public Policy at the Lee Kuan Yew School of Public Policy, we fully appreciate this. Experimentation using novel tools such as product and policy prototyping as part of the “People-centric Approaches to Algorithmic Explainability” project driven by Meta’s TTC Labs and Open Loop presented an opportunity to explore experimental methodologies in the space of emerging technology. What’s more, in a critical area like artificial intelligence (AI) which is already part of the fabric of modern life yet is growing more complex, requires careful consideration of governance approaches.
As academic observers of the project from a public policy perspective, we asked whether and how policy prototyping can be used to effectively inform and support AI governance. Such effectiveness would depend on how learnings are integrated into the formal policy process. To this end, we asked ourselves three key questions:
-
How can policy prototyping be used to inform AI governance in Singapore?
-
What are the conditions of success needed for policy prototyping to be effective?
-
What are the challenges for policy prototyping?
Methodology-wise, we used a case study approach where we utilized desktop research, participant observation through the Design Jam and Policy Prototyping sessions, and in-depth interviews with participants, regulators, and academics.
Conditional factors that enable successful policy prototyping
Our analysis can be broken down into three key areas for policy prototyping: conditions for success, strengths, and challenges. In order for policy prototyping to be successful, there are three conditions identified:
-
Willingness for policy experimentation. This is especially true since AI is a constantly evolving field that requires governance sensitive to industry needs.
-
Technical expertise. This means amassing knowledge in diverse fields in both the public and private sectors to attain unique insights.
-
Active participation. Policy prototyping is reliant on the meaningful participation of businesses and policy makers; it is through such participation that insights are derived and can be used as data points in separate formal policy making processes.
Leveraging strengths and resolving challenges through policy prototyping
Based on our analysis, policy prototyping allows for interactive, inclusive, and adaptive engagements between startups and regulators. This creates a critical communication channel, as one of our interviewees observed, “It’s important to hear the voice of the small companies and small solutions and not just the big companies and big solutions”. This allows regulators to gain diverse perspectives on the challenges that small companies face, which are important data points to consider.
Policy prototyping generates and validates multiple policy options quickly. It integrates diverse stakeholders together to learn from each other through an iterative process. Multiple data sources strengthen the feasibility of ideas, which benefits AI governance guidelines, especially the ones that need to span across multiple sectors that continue to evolve over time.
However, there are challenges to the current policy prototyping approach:
-
Limited stakeholder diversity curtails the level of insight attained. There was a lack of policy stakeholders and developers or data science practitioners due to resourcing and scheduling constraints, which does not reflect the fulsome realities of developing AI-powered services. There was also a lack of consumer representation which indicates a need to change the selection process for non-startup stakeholders.
-
Greater risks associated with policy development versus product development. When it comes to product and policy prototyping, there is more responsibility tied to the latter. The differentiated risks between policy and product development may limit the transferability of learnings between product and policy prototyping. It may also impact how open regulators can be, as they are used to approaching policy with caution.
-
Risk of reducing AI principles to mere compliance components. It is important to deep-dive on how such principles can translate into products and policy. Otherwise, we run the risk of reinforcing a box-ticking approach often taken in response to compliance. To mitigate such risks, intersectional design is needed, as well as more space to discuss ongoing friction between AI principles and the digital ethics culture.
Recommendations to maximize the potential of policy prototyping
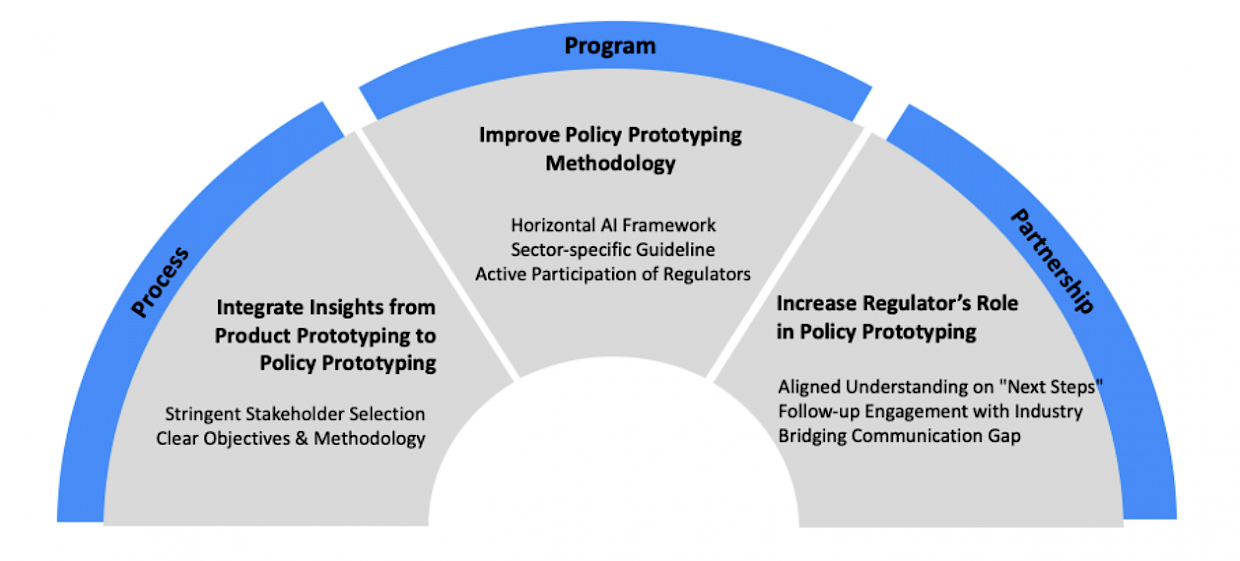
The synthesis of our findings have led us to three themes of recommendations to be considered for policy prototyping: Process, Program, and Partnership.
First, upgrading the process by integrating the strengths of product prototyping into policy prototyping. This requires introducing new angles and viewpoints by having data scientists, consumer welfare groups, and digital rights experts participate in policy prototyping. Policy prototyping workshops can also be better tailored to meet policymakers' interests, by 1) utilizing AI governance frameworks specific to policymakers’ jurisdictions and 2) having government affairs employees participate from the industry.
Second, improving policy prototyping program design. Policy prototyping workshops should host diverse participants from different archetypes, industries, and jurisdictions to develop a broad but strong horizontal framework. For industries that need more granularity, industry-specific policy prototyping sessions should be held to create vertical guidelines based on use cases. Regulators should actively participatein policy prototyping, so that industries can better understand where regulators come from, while policy makers can check whether their policy is implementable and practical.
Third, bolstering partnership with regulators. The success of policy prototyping depends on not just the insights gathered, but how they are integrated into the formal policy process. In addition to holding workshops to generate insights, regulators can consider having a longer time frame with workshops that have consistent themes to materialize a policy prototype. In the meantime, regulators should continue engaging with the industry to help them catch up with evolving policy conversations. Engaging with the right intermediaries – such as officers with technical and data expertise in the public sector, and government affairs professionals in the private sector – can go a long way in bridging the communication gap on both sides.
Overall, policy prototyping has its benefits when its process is designed and used at the right stage of the policy making process. It needs to take into account the direct and indirect risks that come with it, to ensure that governance in a highly contested area is not made further complex, and instead centered on people and diversity.
---
The views, opinions and recollections expressed in this piece are the authors’ own as part of their participation in, and observation of, the People-centric approaches to AI Explainability project. They do not necessarily represent the perspectives of Meta, TTC Labs, Open Loop, IMDA, the Lee Kuan Yew School of Public Policy, and the National University of Singapore. This article was the result of a Policy Analysis Exercise project on “Resolving Artificial Intelligence Governance Challenges through Policy Prototyping” that was led by Masters in Public Policy students at the Lee Kuan Yew School of Public Policy, National University of Singapore.